Overview
Our team of dedicated students and researchers focus on innovative solutions to enhance battery performance, safety, and longevity.
Key Research Areas:
Battery Pack Design: We aim to innovate and optimize battery architecture to improve energy density, safety, and cost-effectiveness. We explore novel approaches and configurations to achieve superior performance in automotive applications.
Battery Management Systems: We leverage machine learning and standardized testing protocols to develop advanced battery management systems (BMS). Our goal is to enhance the performance, reliability, and longevity of batteries by accurately monitoring and managing their state of health, charge, power capability, and other operational characteristics.
Aging-Sensitive Control: Our research focuses on developing aging-sensitive control strategies, to ensure effective management of batteries throughout their entire lifecycle, from initial deployment to end-of-life.
Thermal Management in Battery Systems: We investigate innovative cooling techniques, thermal modelling, and the integration of thermal management into battery pack design to ensure optimal operation during extreme conditions and ultra-fast charging.
Our commitment to these research areas drives our mission to push the boundaries of battery technology and contribute to the advancement of electric mobility.
Publications
We invite you to explore our publications and discover the impactful work our researchers are undertaking to drive the future of energy storage and electric mobility.
Battery SOx and Modelling
Expandable List
Title |
Authors |
Publisher |
Year |
State of charge estimation of lithium-ion batteries: Comparison of GRU, LSTM, and temporal convolutional deep neural networks | Naguib, Mina; Kollmeyer, Phillip J; Emadi, Ali; | IEEE Transportation Electrification Conference & Expo (ITEC) | 2023 |
Feedforward and NARX Neural Network Battery State of Charge Estimation with Robustness to Current Sensor Error | Vieira, Romulo Navega; Kollmeyer, Phillip; Naguib, Mina; Emadi, Ali; | IEEE Transportation Electrification Conference & Expo (ITEC) | 2023 |
A blind modeling tool for standardized evaluation of battery state of charge estimation algorithms | Kollmeyer, Phillip J; Naguib, Mina; Khanum, Fauzia; Emadi, Ali; | IEEE Transportation Electrification Conference & Expo (ITEC) | 2022 |
Battery dual extended Kalman filter state of charge and health estimation strategy for traction applications | Duque, Josimar; Kollmeyer, Phillip J; Naguib, Mina; Emadi, Ali; | IEEE Transportation Electrification Conference & Expo (ITEC) | 2022 |
Lithium-ion battery pack robust state of charge estimation, cell inconsistency, and balancing | Naguib, Mina; Kollmeyer, Phillip; Emadi, Ali; | IEEE Access | 2021 |
A Kalman filter based battery state of charge estimation MATLAB function | Khanum, Fauzia; Louback, Eduardo; Duperly, Federico; Jenkins, Colleen; Kollmeyer, Phillip J; Emadi, Ali; | IEEE Transportation Electrification Conference & Expo (ITEC) | 2021 |
Robust xev battery state-of-charge estimator design using a feedforward deep neural network | Vidal, Carlos; Kollmeyer, Phillip; Naguib, Mina; Malysz, Pawel; Gross, Oliver; Emadi, Ali; | SAE International Journal of Advances and Current Practices in Mobility | 2020 |
Machine learning applied to electrified vehicle battery state of charge and state of health estimation: State-of-the-art | Vidal, Carlos; Malysz, Pawel; Kollmeyer, Phillip; Emadi, Ali; | IEEE Access | 2020 |
Li-ion battery state of charge estimation using long short-term memory recurrent neural network with transfer learning | Vidal, Carlos; Kollmeyer, Phillip; Chemali, Ephrem; Emadi, Ali; | IEEE Transportation Electrification Conference and Expo (ITEC) | 2019 |
State-of-charge estimation of Li-ion batteries using deep neural networks: A machine learning approach | Chemali, Ephrem; Kollmeyer, Phillip J; Preindl, Matthias; Emadi, Ali; | Journal of Power Sources | 2018 |
Simultaneous state and parameter estimation of li-ion battery with one state hysteresis model using augmented unscented kalman filter | Biswas, Atriya; Gu, Ran; Kollmeyer, Phil; Ahmed, Ryan; Emadi, Ali; | IEEE Transportation Electrification Conference and Expo (ITEC) | 2018 |
Long short-term memory networks for accurate state-of-charge estimation of Li-ion batteries | Chemali, Ephrem; Kollmeyer, Phillip J; Preindl, Matthias; Ahmed, Ryan; Emadi, Ali; | IEEE Transactions on Industrial Electronics | 2017 |
Coulomb counting state-of-charge algorithm for electric vehicles with a physics-based temperature dependent battery model | Juang, Larry W; Kollmeyer, Phillip J; Zhao, Ruxiu; Jahns, TM; Lorenz, RD; | IEEE Energy Conversion Congress and Exposition (ECCE) | 2015 |
System identification-based lead-acid battery online monitoring system for electric vehicles | Juang, Larry W; Kollmeyer, Phillip J; Jahns, TM; Lorenz, RD; | IEEE Energy Conversion Congress and Exposition | 2010 |
Title |
Authors |
Publisher |
Year |
Lithium-ion Battery State-of-Health Estimation via Histogram Data, Principal Component Analysis, and Machine Learning | Chen, Junran; Kollmeyer, Phillip; Chiang, Fei; Emadi, Ali; | IEEE Transportation Electrification Conference & Expo (ITEC) | 2023 |
A convolutional neural network for estimation of lithium-ion battery state-of-health during constant current operation | Chen, Junran; Manivanan, Manjula; Duque, Josimar; Kollmeyer, Phillip; Panchal, Satyam; Gross, Oliver; Emadi, Ali; | IEEE transportation electrification conference & expo (ITEC) | 2023 |
A convolutional neural network approach for estimation of li-ion battery state of health from charge profiles | Chemali, Ephrem; Kollmeyer, Phillip J; Preindl, Matthias; Fahmy, Youssef; Emadi, Ali; | Energies | 2022 |
Battery dual extended Kalman filter state of charge and health estimation strategy for traction applications | Duque, Josimar; Kollmeyer, Phillip J; Naguib, Mina; Emadi, Ali; | IEEE Transportation Electrification Conference & Expo (ITEC) | 2022 |
Machine learning applied to electrified vehicle battery state of charge and state of health estimation: State-of-the-art | Vidal, Carlos; Malysz, Pawel; Kollmeyer, Phillip; Emadi, Ali; | IEEE Access | 2020 |
Title |
Authors |
Publisher |
Year |
Implementation of online battery state-of-power and state-of-function estimation in electric vehicle applications | Juang, Larry W; Kollmeyer, Phillip J; Jahns, Thomas M; Lorenz, Robert D; | IEEE Energy Conversion Congress and Exposition (ECCE) | 2012 |
Title |
Authors |
Publisher |
Year |
Sequence training and data shuffling to enhance the accuracy of recurrent neural network based battery voltage models | Chen, Junran; Kollmeyer, Phillip; Panchal, Satyam; Masoudi, Yasaman; Gross, Oliver; Emadi, Ali; | SAE World Congress | 2024 |
Estimating battery state of charge using recurrent and non-recurrent neural networks | Vidal, Carlos; Malysz, Pawel; Naguib, Mina; Emadi, Ali; Kollmeyer, Phillip J; | Journal of Energy Storage | 2022 |
Robust data-driven battery state of charge estimation for hybrid electric vehicles | Feraco, Stefano; Anselma, Pier Giuseppe; Bonfitto, Angelo; Kollmeyer, Phillip J; | SAE International Journal of Electrified Vehicles | 2022 |
A comparative study between physics, electrical and data driven lithium-ion battery voltage modeling approaches | Liang, Yang; Emadi, Ali; Gross, Oliver; Vidal, Carlos; Canova, Marcello; Panchal, Satyam; Kollmeyer, Phillip; Naguib, Mina; Khanum, Fauzia; | SAE World Congress | 2022 |
Comparative study between equivalent circuit and recurrent neural network battery voltage models | Naguib, Mina; Vidal, Carlos; Kollmeyer, Phillip; Malysz, Pawel; Gross, Oliver; Emadi, Ali; | SAE World Congress | 2021 |
xEV Li-ion battery low-temperature effects | Vidal, Carlos; Gross, Oliver; Gu, Ran; Kollmeyer, Phillip; Emadi, Ali; | IEEE transactions on vehicular technology | 2019 |
A compact methodology via a recurrent neural network for accurate equivalent circuit type modeling of lithium-ion batteries | Zhao, Ruxiu; Kollmeyer, Phillip J; Lorenz, Robert D; Jahns, Thomas M; | IEEE Transactions on Industry Applications | 2018 |
Li-ion battery model performance for automotive drive cycles with current pulse and EIS parameterization | Kollmeyer, Phillip; Hackl, Andreas; Emadi, Ali; | IEEE transportation electrification conference and expo (ITEC) | 2017 |
A compact unified methodology via a recurrent neural network for accurate modeling of lithium-ion battery voltage and state-of-charge | Zhao, Ruxiu; Kollmeyer, Phillip J; Lorenz, Robert D; Jahns, Thomas M; | IEEE Energy Conversion Congress and Exposition (ECCE) | 2017 |
Modeling of second-life batteries for use in a CERTS microgrid | Hart, PJ; Kollmeyer, PJ; Juang, LW; Lasseter, RH; Jahns, TM; | Power and Energy Conference at Illinois (PECI) | 2014 |
Improved modeling of lithium-based batteries using temperature-dependent resistance and overpotential | Juang, Larry W; Kollmeyer, Phillip J; Jahns, TM; Lorenz, RD; | IEEE Transportation Electrification Conference and Expo (ITEC) | 2014 |
The impact of DC bias current on the modeling of lithium iron phosphate and lead-acid batteries observed using electrochemical impedance spectroscopy | Juang, Larry W; Kollmeyer, Phillip J; Zhao, Ruxiu; Jahns, TM; Lorenz, RD; | IEEE Energy Conversion Congress and Exposition (ECCE) | 2014 |
Improved nonlinear model for electrode voltage–current relationship for more consistent online battery system identification | Juang, Larry W; Kollmeyer, Phillip J; Jahns, Thomas M; Lorenz, Robert D; | IEEE Transactions on Industry Applications | 2013 |
Title |
Authors |
Publisher |
Year |
Application of deep neural networks for lithium-ion battery surface temperature estimation under driving and fast charge conditions | Naguib, Mina; Kollmeyer, Phillip; Emadi, Ali; | IEEE Transactions on Transportation Electrification | 2022 |
Accurate surface temperature estimation of lithium-ion batteries using feedforward and recurrent artificial neural networks | Naguib, Mina; Kollmeyer, Phillip; Vidal, Carlos; Emadi, Ali; | IEEE Transportation Electrification Conference & Expo (ITEC) | 2021 |
Title |
Authors |
Publisher |
Year |
Estimating battery state of charge using recurrent and non-recurrent neural networks | Vidal, Carlos; Malysz, Pawel; Naguib, Mina; Emadi, Ali; Kollmeyer, Phillip J; | Journal of Energy Storage | 2022 |
Microprocessor execution time and memory use for battery state of charge estimation algorithms | Naguib, Mina; Kollmeyer, Phillip; Vidal, Carlos; Duque, Josimar; Gross, Oliver; Emadi, Ali; | SAE World Congress | 2022 |
Battery Aging
Expandable List
Title |
Authors |
Publisher |
Year |
Battery state-of-health adaptive energy management of hybrid electric vehicles | Anselma, Pier Giuseppe; Kollmeyer, Phillip; Emadi, Ali; | IEEE Transportation Electrification Conference & Expo (ITEC) | 2022 |
Economic Payback Time of Battery Pack Replacement for Hybrid and Plug-In Hybrid Electric Vehicles | Anselma, Pier Giuseppe; Kollmeyer, Phillip J; Feraco, Stefano; Bonfitto, Angelo; Belingardi, Giovanni; Emadi, Ali; Amati, Nicola; Tonoli, Andrea; | IEEE Transactions on Transportation Electrification | 2022 |
Battery state-of-health sensitive energy management of hybrid electric vehicles: Lifetime prediction and ageing experimental validation | Anselma, Pier Giuseppe; Kollmeyer, Phillip; Lempert, Jeremy; Zhao, Ziyu; Belingardi, Giovanni; Emadi, Ali; | Applied Energy | 2021 |
Assessing impact of heavily aged batteries on hybrid electric vehicle fuel economy and drivability | Anselma, Pier Giuseppe; Kollmeyer, Phillip J; Feraco, Stefano; Bonfitto, Angelo; Belingardi, Giovanni; Emadi, Ali; Amati, Nicola; Tonoli, Andrea; | IEEE Transportation Electrification Conference & Expo (ITEC) | 2021 |
Multitarget Evaluation of Hybrid Electric Vehicle Powertrain Architectures Considering Fuel Economy and Battery Lifetime | Anselma, Pier Giuseppe; Kollmeyer, Phillip; Belingardi, Giovanni; Emadi, Ali; | SAE World Congress | 2020 |
Multi-objective hybrid electric vehicle control for maximizing fuel economy and battery lifetime | Anselma, Pier Giuseppe; Kollmeyer, Phillip; Belingardi, Giovanni; Emadi, Ali; | IEEE Transportation Electrification Conference & Expo (ITEC) | 2020 |
Battery Thermal Management and Hybrid Energy Storage
Expandable List
Title |
Authors |
Publisher |
Year |
Review of Production Electric Vehicle Battery Thermal Management Systems and Experimental Testing of a Production Battery Module | Uwalaka, Lucia Ifunanya; Yao, Qi; Kollmeyer, Phillip; Emadi, Ali; | SAE World Congress | 2024 |
3D FEA thermal modeling with experimentally measured loss gradient of large format ultra-fast charging battery module used for EVs | Zhao, Ziyu; Panchal, Satyam; Kollmeyer, Phillip; Emadi, Ali; Gross, Oliver; Dronzkowski, David; Mahajan, Vishal; David, Lamuel; | SAE World Congress | 2022 |
Cell selection and thermal management system design for a 5C-rate ultrafast charging battery module | Lempert, Jeremy; Kollmeyer, Phillip J; He, Melissa; Haußmann, Mike; Cotton, James S; Emadi, Ali; | Journal of Power Sources | 2022 |
Experimental Comparison of Two Liquid Cooling Methods for Ultrafast Charging Lithium-Ion Battery Modules | Zhao, Ziyu; Kollmeyer, Phillip J; Lempert, Jeremy M; Emadi, Ali; | IEEE Transportation Electrification Conference & Expo (ITEC) | 2021 |
Battery entropic heating coefficient testing and use in cell-level loss modeling for extreme fast charging | Lempert, Jeremy; Kollmeyer, Phillip; Malysz, Pawel; Gross, Oliver; Cotton, James; Emadi, Ali; | SAE International Journal of Advances and Current Practices in Mobility | 2020 |
A Comparison of the Performance and Thermal Management Requirements of Lithium-Ion batteries During Ultra-Fast Charging | He, Melissa S; Kollmeyer, Phillip J; Haußmann, Mike; Emadi, Ali; | IEEE Transportation Electrification Conference and Expo (ITEC) | 2018 |
Title |
Authors |
Publisher |
Year |
Real-time control of a full scale Li-ion battery and Li-ion capacitor hybrid energy storage system for a plug-in hybrid vehicle | Kollmeyer, Phillip J; Wootton, Mackenzie; Reimers, John; Opila, Daniel F; Kurera, Himesh; Kadakia, Mrudang; Gu, Ran; Stiene, Tyler; Chemali, Ephrem; Wood, Megan; | IEEE Transactions on Industry Applications | 2019 |
Optimized operation of a hybrid energy storage system with lto batteries for high power electrified vehicles | Nemeth, Thomas; Kollmeyer, Phillip J; Emadi, Ali; Sauer, Dirk Uwe; | IEEE Transportation Electrification Conference and Expo (ITEC) | 2019 |
Optimal performance of a full scale li-ion battery and li-ion capacitor hybrid energy storage system for a plug-in hybrid vehicle | Kollmeyer, Phillip; Wootton, Mackenzie; Reimers, John; Stiene, Tyler; Chemali, Ephrem; Wood, Megan; Emadi, Ali; | IEEE Energy Conversion Congress and Exposition (ECCE) | 2017 |
Modeling of low-temperature operation of a hybrid energy storage system with a Butler-Volmer equation based battery model | Kollmeyer, Phillip J; Shridar, Anantharaghavan; Jahns, TM; | IEEE Energy Conversion Congress and Exposition (ECCE) | 2016 |
Model-based control design for a battery/ultracapacitor DC-DC converter system | Sridhar, Anantharaghavan; Kollmeyer, Phillip J; Jahns, TM; | IEEE Energy Conversion Congress and Exposition (ECCE) | 2016 |
Loss optimization and ultracapacitor pack sizing for vehicles with battery/ultracapacitor hybrid energy storage | Kollmeyer, Phillip J; Juang, Larry W; Jahns, TM; | IEEE Transportation Electrification Conference and Expo (ITEC) | 2014 |
Electric Vehicle and Powertrain Optimization
Expandable List
Title |
Authors |
Publisher |
Year |
A Review of Production Multi-Motor Electric Vehicles and Energy Management and Model Predictive Control Techniques | Allca-Pekarovic, Alexander; Kollmeyer, Phillip; Middleton, Mairi; Emadi, Ali; | SAE World Congress | 2024 |
Single-versus Dual-Motor Battery Electric Vehicles Energy Consumption Comparison Across Payloads and Cycles | Louback, Eduardo; Kollmeyer, Phillip J; Machado, Fabricio A; Allca-Pekarovic, Alexander; Shiguemoto, Eduardo Akira; Dilevrano, Gaetano; Emadi, Ali; | IEEE Transportation Electrification Conference and Expo (ITEC) | 2024 |
Loss modeling and testing of 800 V DC bus IGBT and SiC traction inverter modules | Allca-Pekarovic, Alexander; Kollmeyer, Phillip J; Reimers, John; Mahvelatishamsabadi, Parisa; Mirfakhrai, Tissaphern; Naghshtabrizi, Payam; Emadi, Ali; | IEEE Transactions on Transportation Electrification | 2023 |
Comparison of igbt and sic inverter loss for 400v and 800v dc bus electric vehicle drivetrains | Allca-Pekarovic, Alexander; Kollmeyer, Phillip J; Mahvelatishamsabadi, Parisa; Mirfakhrai, Tissa; Naghshtabrizi, Payam; Emadi, Ali; | IEEE Energy Conversion Congress and Exposition (ECCE) | 2020 |
800-V electric vehicle powertrains: Review and analysis of benefits, challenges, and future trends | Aghabali, Iman; Bauman, Jennifer; Kollmeyer, Phillip J; Wang, Yawei; Bilgin, Berker; Emadi, Ali; | IEEE Transactions on Transportation Electrification | 2020 |
Development and implementation of a battery-electric light-duty class 2a truck including hybrid energy storage | Kollmeyer, Phillip J; | PhD Dissertation | 2015 |
Design of an electric powertrain for a Ford F150 crew cab truck utilizing a lithium battery pack and an interior PM synchronous machine drive | Kollmeyer, Phillip J; Lamb, Will; Juang, Larry W; McFarland, James D; Jahns, TM; Sarlioglu, B; | IEEE Transportation Electrification Conference and Expo (ITEC) | 2012 |
Evaluation of an electromechanical model for a Corbin Sparrow electric vehicle | Kollmeyer, P.J.; Juang, L.W.; Jahns, T.M.; | Vehicle Power and Propulsion Conference (VPPC) | 2011 |
Development of an electromechanical model for a Corbin Sparrow electric vehicle | Kollmeyer, Phillip J; Juang, Larry W; Jahns, TM; | IEEE Vehicle Power and Propulsion Conference (VPPC) | 2011 |
Title |
Authors |
Publisher |
Year |
Analytical loss model for single-and two-speed electric vehicle gearboxes | Machado, Fabricio; Kollmeyer, Phillip J; Emadi, Ali; | IEEE Transportation Electrification Conference and Expo, Asia-Pacific (ITEC Asia-Pacific) | 2023 |
Chevrolet bolt electric vehicle model validated with on-the-road data and applied to estimating the benefits of a multi-speed gearbox | Machado, Fabricio; Kollmeyer, Phillip; Emadi, Ali; | SAE World Congress | 2022 |
Real-time performance and driveability analysis of a clutchless multi-speed gearbox for battery electric vehicle applications | Louback, Eduardo; Machado, Fabricio; Bruck, Lucas; Kollmeyer, Phillip J; Emadi, Ali; | IEEE Transportation Electrification Conference & Expo (ITEC) | 2022 |
Multi-speed gearboxes for battery electric vehicles: Current status and future trends | Machado, Fabricio A; Kollmeyer, Phillip J; Barroso, Daniel G; Emadi, Ali; | IEEE Open Journal of Vehicular Technology | 2021 |
Comparison of class 2a truck electric vehicle drivetrain losses for single-and two-speed gearbox systems with IPM traction machines | Kollmeyer, PJ; McFarland, JD; Jahns, TM; | IEEE International Electric Machines & Drives Conference (IEMDC) | 2015 |
Title |
Authors |
Publisher |
Year |
Experimental Characterization and Modeling of a YASA P400 Axial Flux PM Traction Machine for Performance Analysis of a Chevy Bolt EV | Allca-Pekarovic, Alexander; Kollmeyer, Phillip J; Forsyth, Alexander; Emadi, Ali; | IEEE Transactions on Industry Applications | 2023 |
Experimental characterization and modeling of a YASA P400 axial flux PM traction machine for electric vehicles | Allca-Pekarovic, Alexander; Kollmeyer, Phillip J; Forsyth, Alexander; Emadi, Ali; | IEEE Transportation Electrification Conference & Expo (ITEC) | 2022 |
Title |
Authors |
Publisher |
Year |
Loss modeling and testing of 800 V DC bus IGBT and SiC traction inverter modules | Allca-Pekarovic, Alexander; Kollmeyer, Phillip J; Reimers, John; Mahvelatishamsabadi, Parisa; Mirfakhrai, Tissaphern; Naghshtabrizi, Payam; Emadi, Ali; | IEEE Transactions on Transportation Electrification | 2023 |
Comparison of igbt and sic inverter loss for 400v and 800v dc bus electric vehicle drivetrains | Allca-Pekarovic, Alexander; Kollmeyer, Phillip J; Mahvelatishamsabadi, Parisa; Mirfakhrai, Tissa; Naghshtabrizi, Payam; Emadi, Ali; | IEEE Energy Conversion Congress and Exposition (ECCE) | 2020 |
Onboard unidirectional automotive G2V battery charger using sine charging and its effect on li-ion batteries | Prasad, Rashmi; Namuduri, Chandra; Kollmeyer, Phillip; | IEEE Energy Conversion Congress and Exposition (ECCE) | 2015 |
Model-based control design for a battery/ultracapacitor DC-DC converter system | Sridhar, Anantharaghavan; Kollmeyer, Phillip J; Jahns, TM; | IEEE Energy Conversion Congress and Exposition (ECCE) | 2016 |
V2G integration and experimental demonstration on a lab-scale microgrid | Mendoza-Araya, Patricio A; Kollmeyer, Phillip J; Ludois, Daniel C; | IEEE Energy Conversion Congress and Exposition (ECCE) | 2013 |
Dataset and Algorithms
Our commitment to advancing battery technology and electric vehicles is reflected in the high-quality, open-source datasets we provide. This section is dedicated to providing access to the datasets and algorithms that underpin our research.
Expandable List
TITLE |
YEAR |
LG E66 Prismatic 66 Ahhttps://doi.org/10.5683/SP3/7JP3NM Borealis Data |
2024 |
Tesla Model 3 21700 4.9 Ahhttps://doi.org/10.5683/SP3/ZVTR4B Borealis Data |
2023 |
SB LiMotive Prismatic 5 Ahhttps://doi.org/10.5683/SP3/LFPKAS Borealis Data |
2023 |
LG HG2 18650 3.0 Ahhttps://data.mendeley.com/datasets/b5mj79w5w9/2 Mendeley Data |
2020 |
Samsung 30T 21700 3.0 Ahhttps://data.mendeley.com/datasets/9xyvy2njj3/1 Mendeley Data |
2020 |
Turnigy Pouch 5.0 Ahhttps://data.mendeley.com/datasets/4fx8cjprxm/1 Mendeley Data |
2020 |
Panasonic NCR18650PF 2.9 Ahhttps://data.mendeley.com/datasets/wykht8y7tg/1 Mendeley Data |
2018 |
TITLE |
YEAR |
Porsche Taycan LG E66 Modulehttps://doi.org/10.5683/SP3/7JP3NM Borealis Data |
2024 |
Samsung 30T 3.0 Ah 21700https://doi.org/10.5683/SP3/UYPYDJ Borealis Data |
2023 |
TITLE |
YEAR |
A123 LFP 2.5 Ah 26650https://doi.org/10.5683/SP3/23MFNE Borealis Data |
2023 |
Samsung 30T 3.0 Ah 21700https://doi.org/10.5683/SP3/UYPYDJ Borealis Data |
2023 |
TITLE |
YEAR |
Convolutional Neural Network (CNN) SOH Estimatorhttps://doi.org/10.5683/SP3/6AGUAW Borealis Data |
2023 |
LSTM and FNN Machine Learning SOC Estimatorshttps://data.mendeley.com/datasets/xf68bwh54v/1 Mendeley |
2021 |
Kalman Filter SOC EstimatorMathworks File Exchange |
2021 |
Vehicles and Student Teams
We are proud to support and collaborate with our student design teams at McMaster University, in the development of advanced battery packs. Our hands-on guidance and state-of-the-art facilities empower students to create high-quality, reliable energy storage solutions that power innovative vehicles.
McMaster Battery Workforce Challenge
The Battery Workforce Challenge is a three-year student competition (2023-2026) that challenges universities and vocational schools from across North America to design, build, test, and integrate an advanced EV battery pack. McMaster University was selected as one of the 12 universities across North America, and one of two universities in Canada, to participate in this competition. You can read more about the McMaster Battery Workforce Challenge team here.
McMaster Solar Car Team
The McMaster Solar Car Team is a student-run organization that designs, constructs, and races solar electric vehicles. McMaster students are able to utilize the state-of-the-art equipment at the battery research labs to design, build, and test the battery pack! You can learn more about the team by visiting their website here.
Ford F150, ICE to EV: Dr. Kollmeyer’s Ph.D. Dissertation
Dr. Kollmery’s Ph.D. thesis at the University of Wisconsin includes the conversion of a Ford F-150 truck from an internal combustion engine (ICE) to an advanced, highly instrumented electric research vehicle (EV). You can read the full specifications of the converted vehicle here and his complete dissertation here.
Corbin Sparrow: Dr. Kollmeyer’s Masters Project
Stellantis Project
Patents
Title |
Authors |
Patent # |
Year |
Mobile medical ventilator | Kollmeyer, Phillip J; Kutko, Stefan I; Tham, Robert; Rick, Norman; Woods, Jonathan L; | US Patent 8,960,193 | 2015 |
Pressure targeted ventilator using an oscillating pump | Kollmeyer, Phillip J; Tham, Robert; Rick, Norman; | US Patent App. 11/461,792 | 2008 |
Battery State of Charge (SOC) Estimator Standardized Testing Tool
There are hundreds of approaches to estimating battery state of charge (SOC), some better suited for specific applications, which makes it very hard to fairly compare results reported in different papers. SOC estimation techniques such as coulomb counting or OCV vs. SOC relationships can be subject to nuances in data and conditions that skew results. Some of these issues include sensor errors, initial value errors, or other complex factors affecting battery performance (e.g., cold weather, cell internal resistance).
This standardized model testing tool is an effective method developed to enable a fair comparison of SOC estimators from various sources and authors, eliminating bias as a result of human and/or external factors by testing models over a wide range of conditions on data which is blinded from the user. The submission process is detailed here, making it convenient for students or researchers to access the standardized model testing tool and open access data and to validate their algorithms.
The initial step is to download the open data here and to create an SOC estimator with the data. For more detailed information see the User’s Guide.
Then follow these procedures to utilize the SOC Estimation Standardized Blind Model Testing Tool :

Expandable List
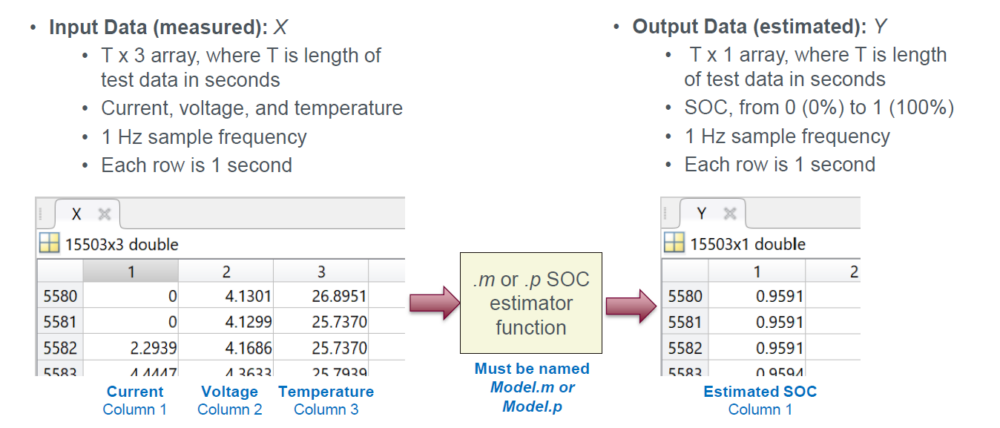
**Create .p version of a .m file by typing ‘pcode model.m’ in the command window
*Learn more about p-code file here.
NOTE: All code submitted to the blind modelling tool will be kept confidential and will only be used to determine results.
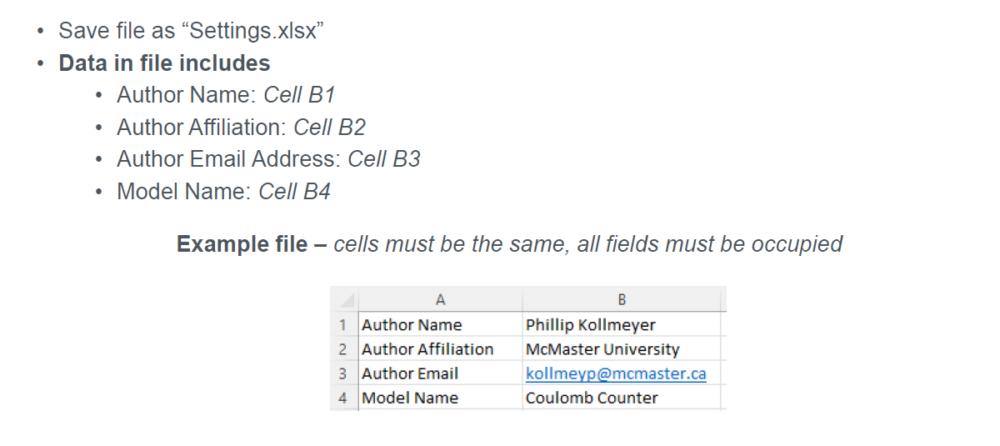
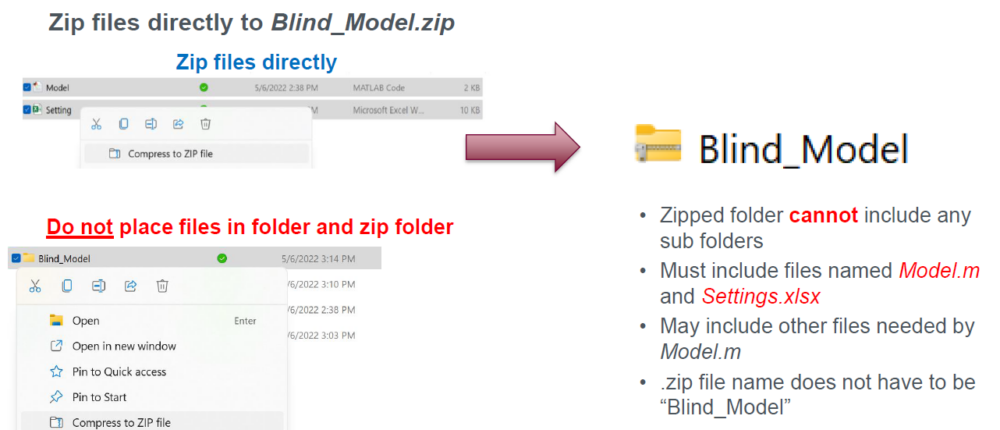
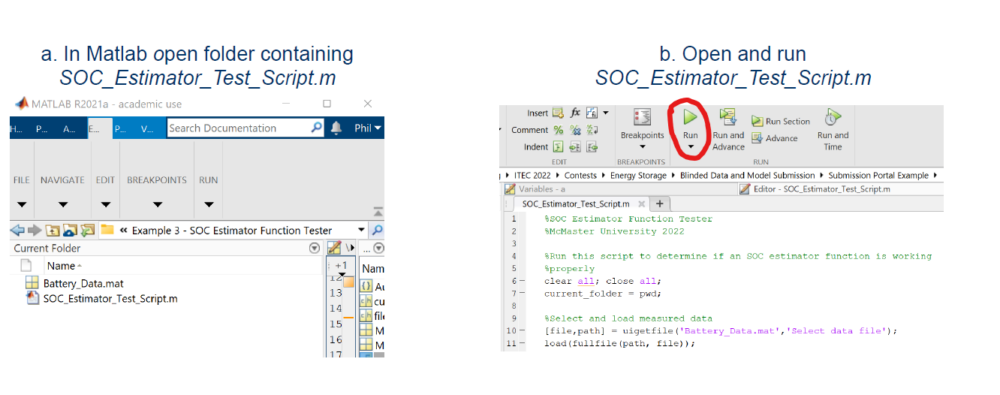
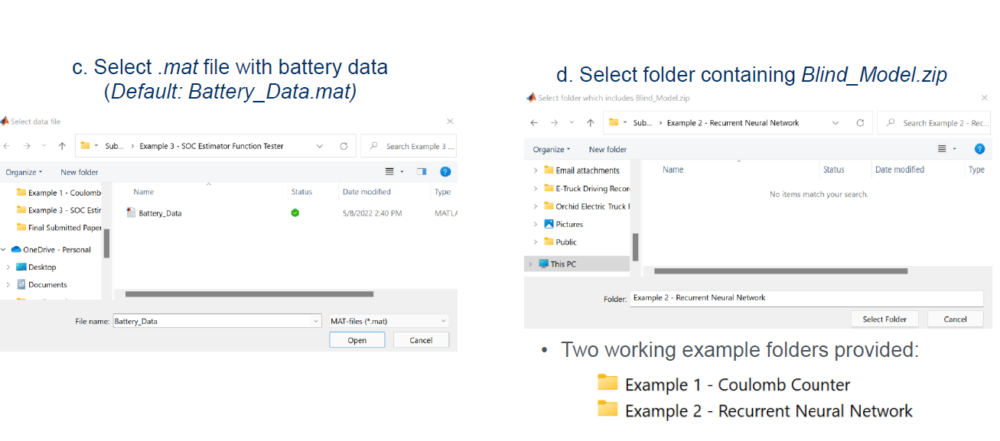
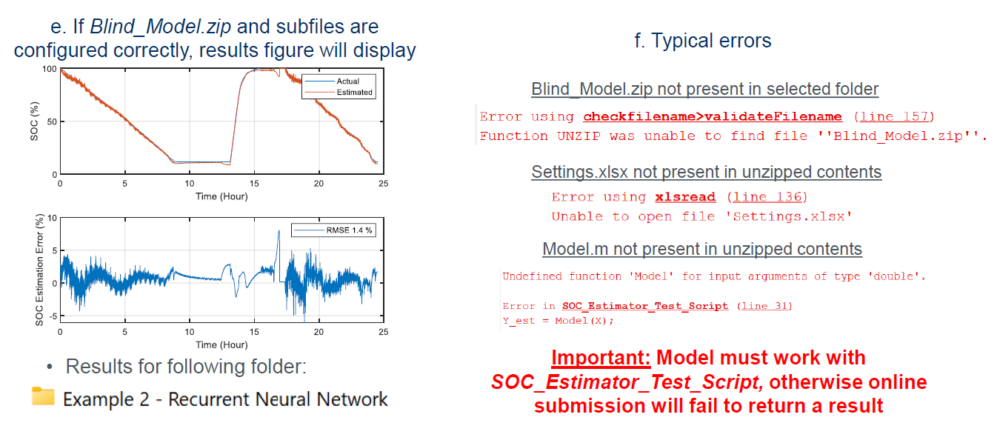
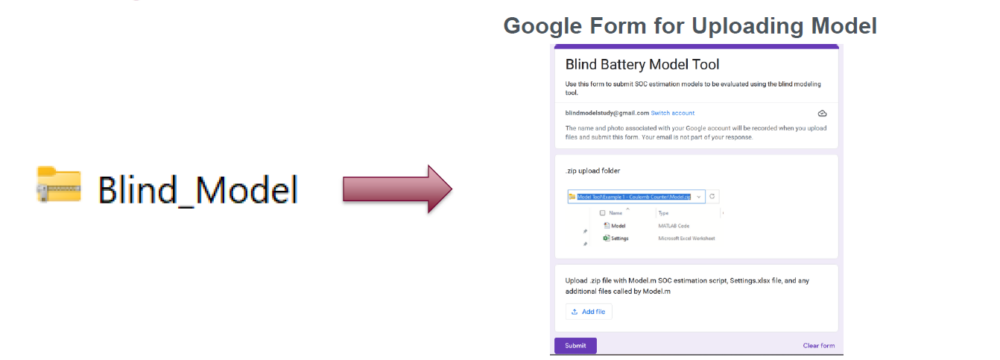
*Access the form here.
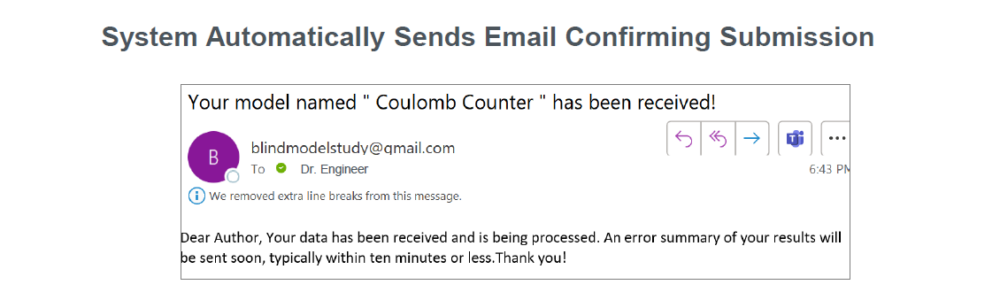
*If email is not received within one hour, the test system is likely down. Send email to Dr. Kollmeyer requesting support.
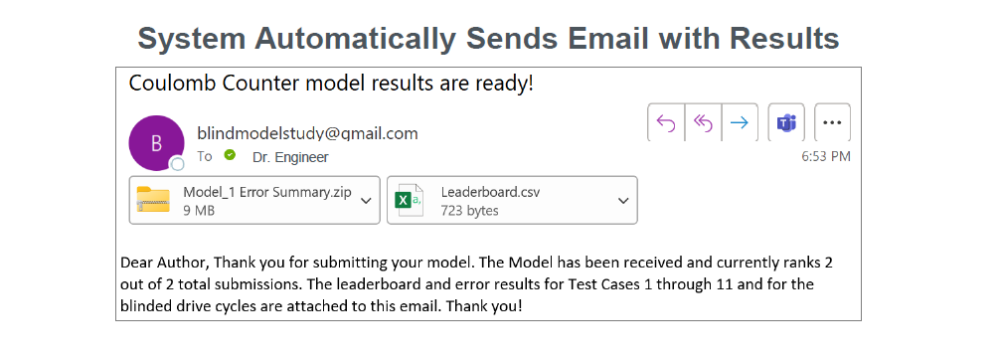
***If email is not received within one hour and confirmation email (step 6) was received, then the model has an error
**Investigate and correct cause of error and re-submit (see step 4)
*If issues cannot be resolved, send email to Dr. Kollmeyer requesting support.
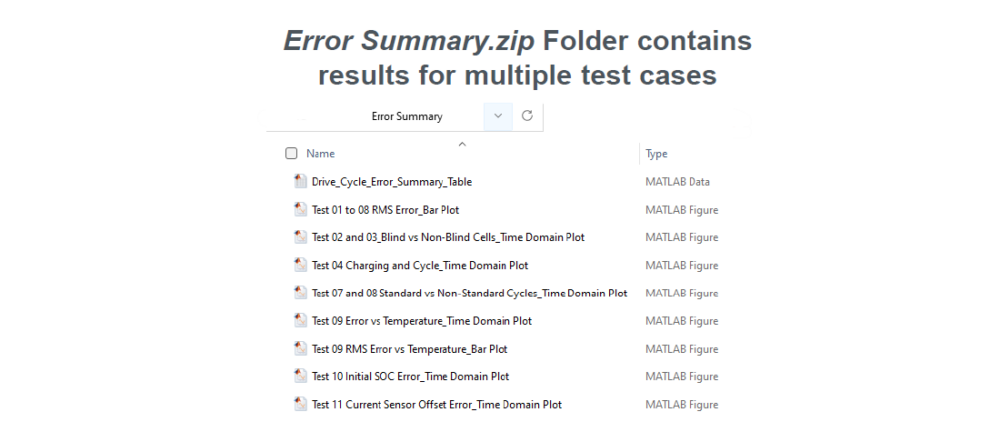
*See ITEC 2022 paper for complete description of test cases: A Blind Modeling Tool for Standardized Evaluation of Battery State of Charge Estimation Algorithms.
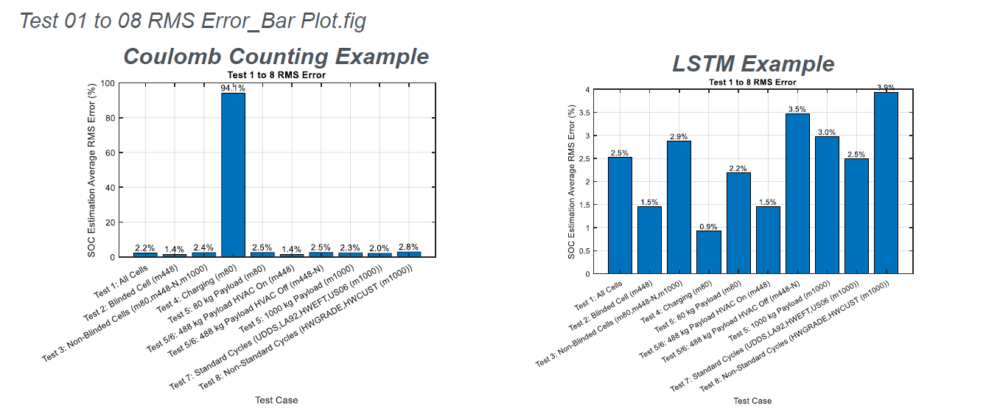
*Charging error high for coulomb counting because charge starts around 0% SOC, and coulomb counting code assumes all test cycles start at 100% SOC.
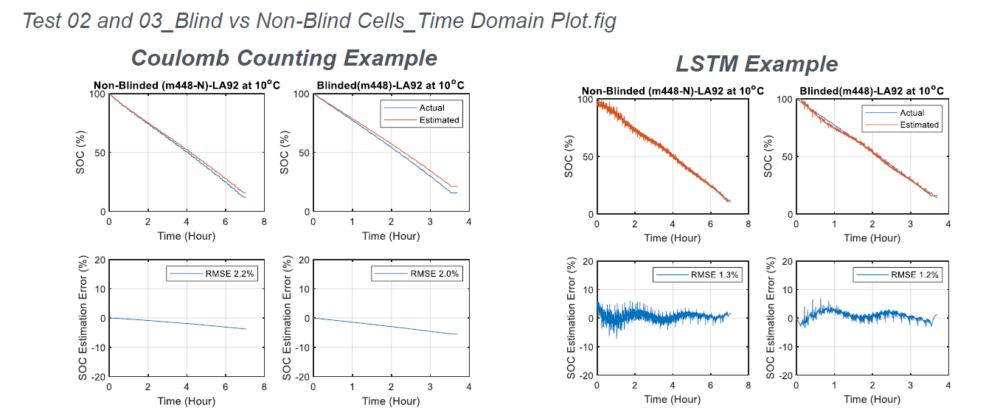
*LTSM more accurate, but somewhat noisy.
*Charging case starts at 0% SOC – causing high error for coulomb counting which assumes 100% SOC at start of file.

*LSTM performs well, except for HWGRADE where high power results in high error.
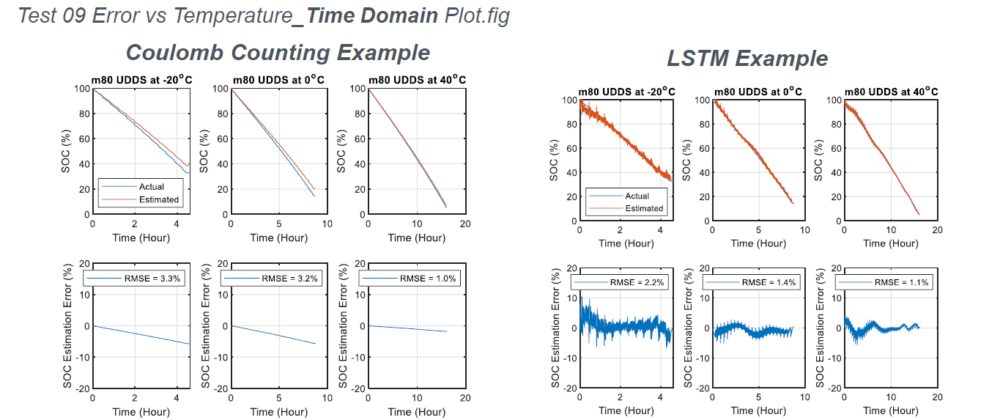
*Coulomb counting error higher at low temperature due to cell aging (cold temperature tests done last) LSTM error higher at low temperature due to nonlinear, higher resistance of cell at low temperatures.
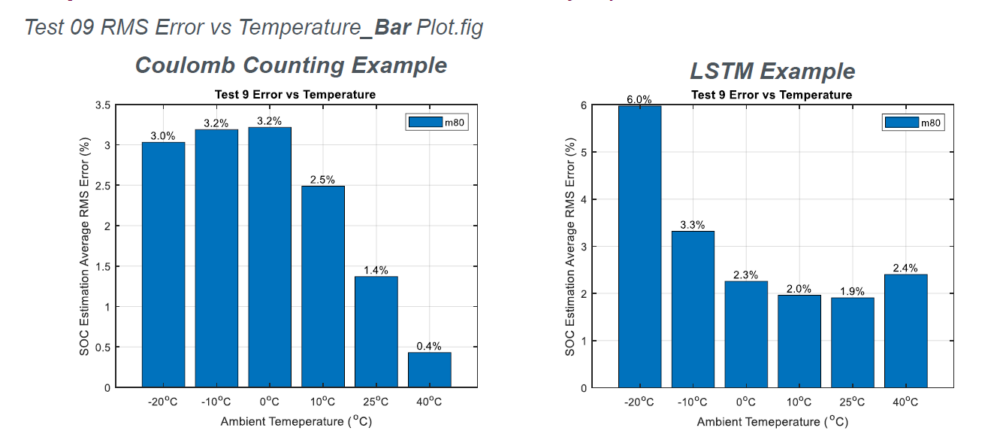
*Coulomb counting error higher at low temperature due to cell aging (cold temperature tests done last) LSTM error higher at low temperature due to nonlinear, higher resistance of cell at low temperatures.
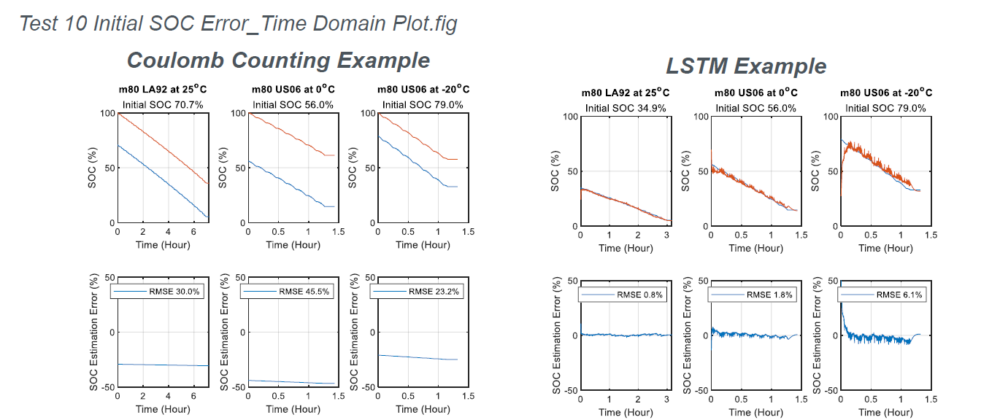
*Coulomb counting can’t adjust to initial SOC (assumes 100% at start of file) LSTM is able estimate the correct SOC value.

*Coulomb counter integrates current sensor error, LSTM handles offset fairly well at higher temperatures.

*Drive cycle error values can be used for making general comparisons and finding trouble cases (high max error, etc.).
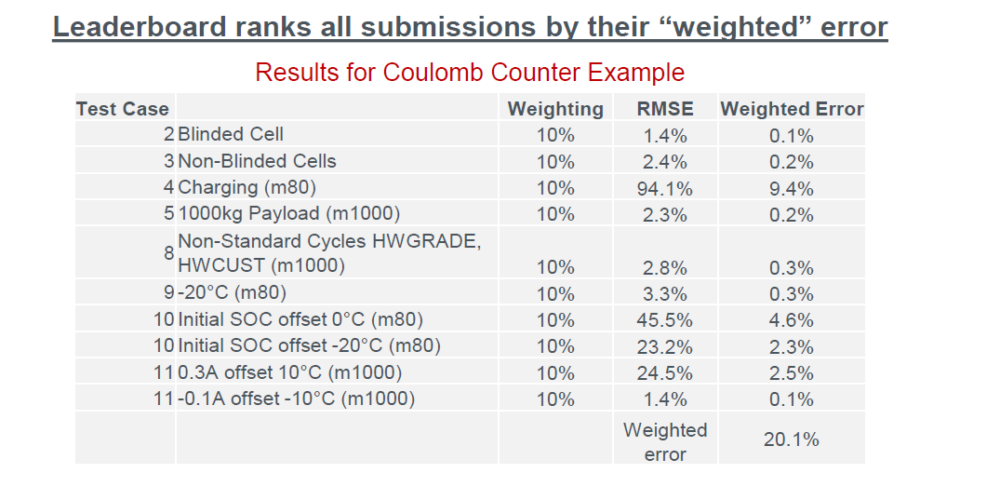
